Empowering R&D with Data Analysis and Uncertainty Quantification
Uptimai is a data analysis and optimization software that helps engineering teams in the auto and aerospace industries make design decisions faster and more accurately by using AI to evaluate even small datasets and present results using modern visualisation tools.
How it works
Your non-optimized design
Your products and designs are modern but highly complex with many dependencies or lots of data types. Moreover, design aspects are multidisciplinary and such that it leads to trade-off which is not optimal.
Coupling
Couple Uptimai software with your simulation platform (Nastran, Ansys, Abaqus…) for enhancement of your simulation processes, use your data directly or combine both simulation and measurement directly.
Computing
The Uptimai Machine Learning (ML) algorithm builds an accurate model based on your data or simulation for fast and reliable processing. Uptimai ML models are extremely effective (e.g. 16-dimensional problem for 50 samples) and can handle even the most complex issues.
Graphical results
From the created model and thanks to our post-processing tools, you can obtain deeper insights needed to optimize your design. Combine simulation with real data measurements, find anomalies in your data, do an optimization which takes into account experts' opinions and many more …
Case studies

Retainer Optimization
Design, analysis and optimization of retainer materials
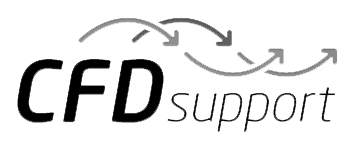
Centrifugal Fan
Turbomachinery CFD study
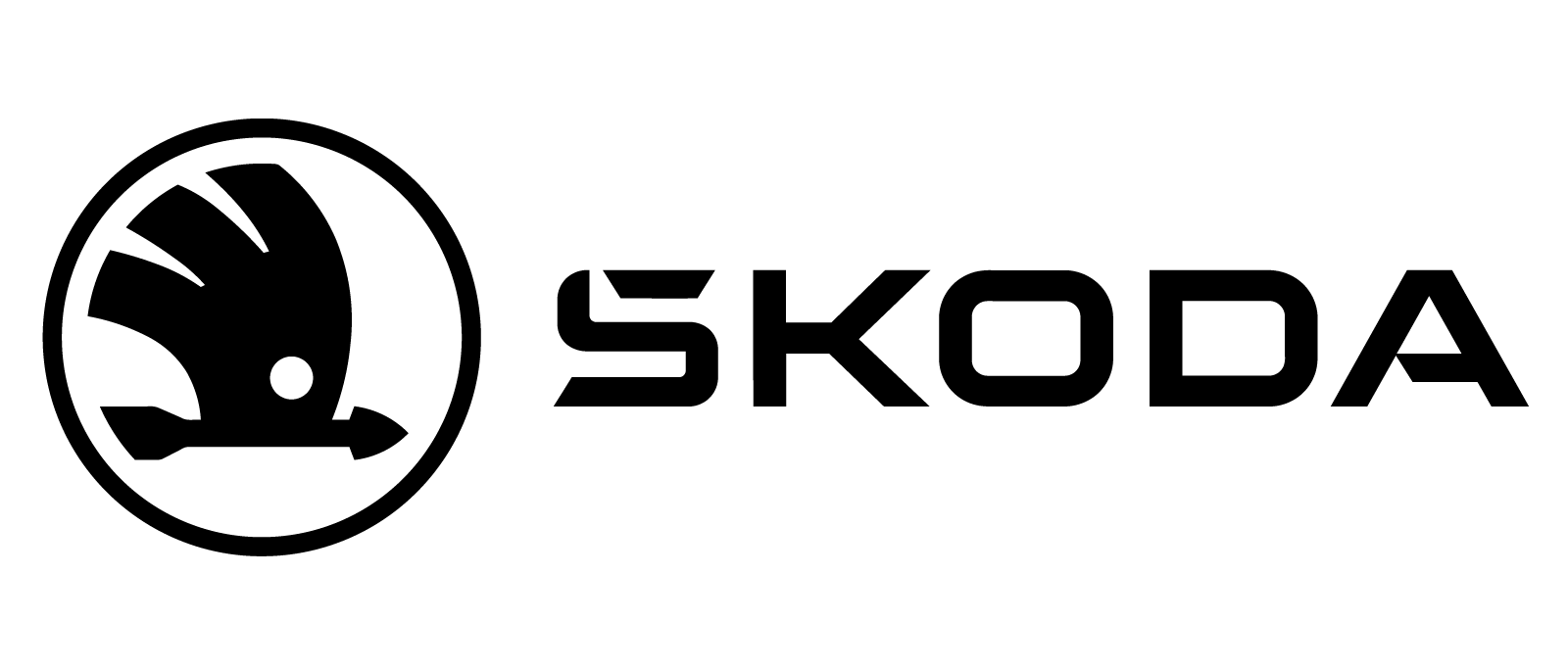
Car Box Beam Deformation
Absorbed impact energy increased by 10%
Testimonials
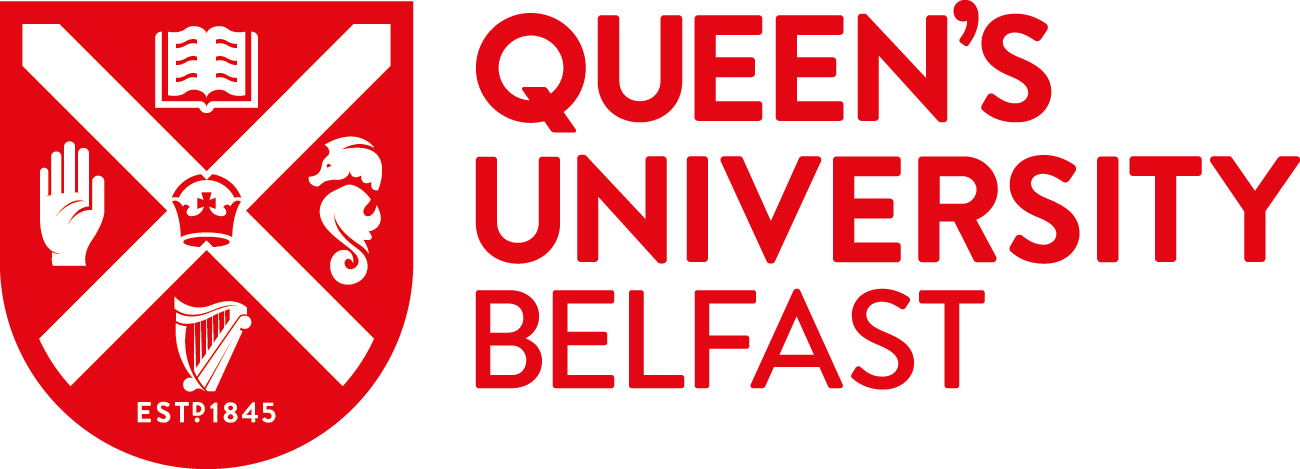
Partners
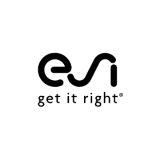
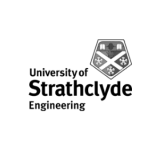
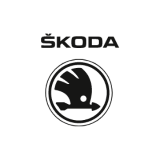
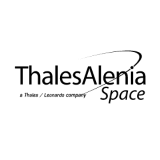
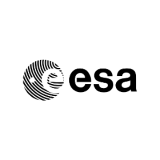
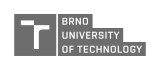
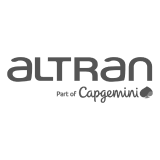
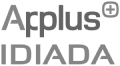